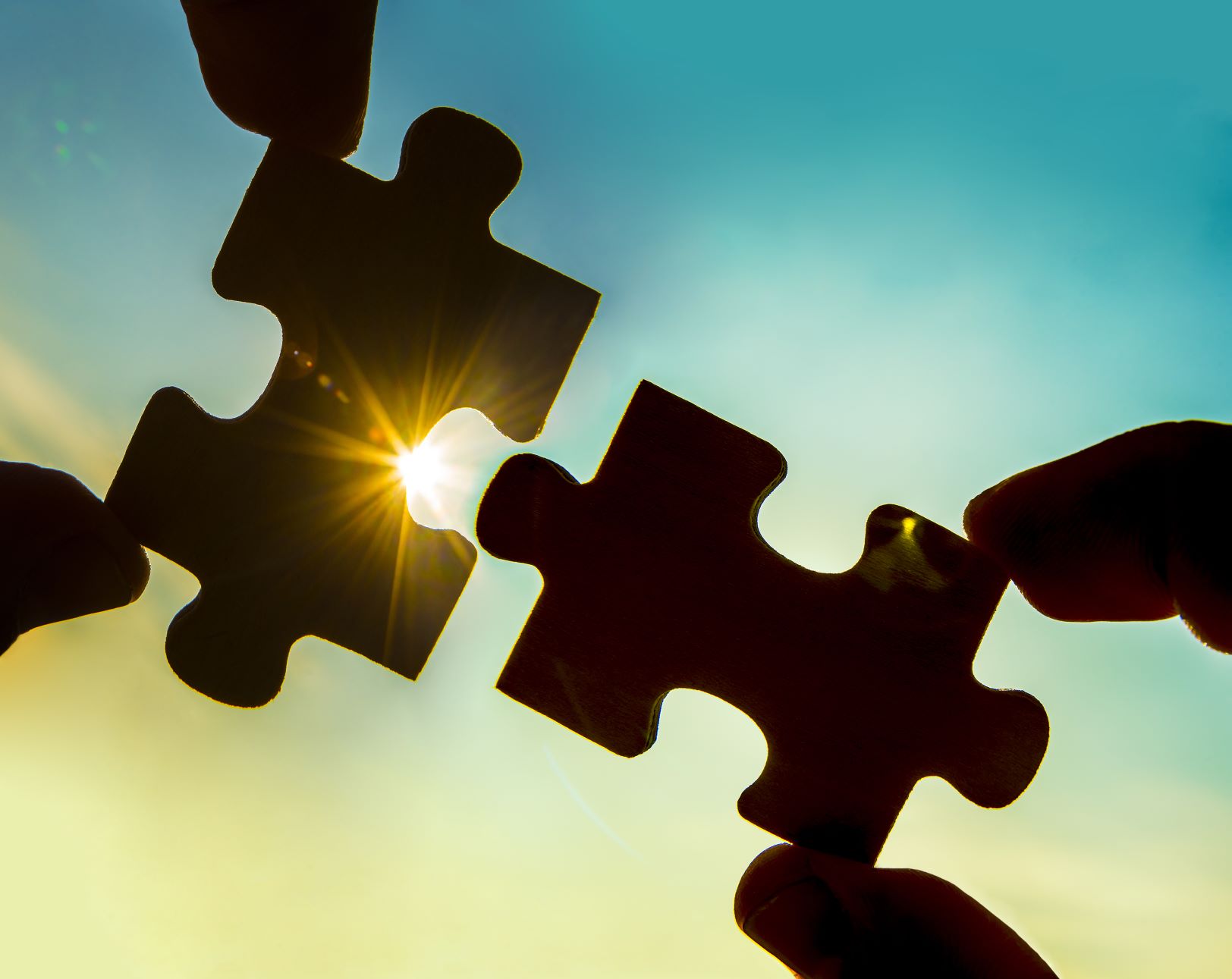
The Frontier of Energy Analytics: Prescriptive Analytics (A Step Beyond Descriptive)
For years energy analytics has focused on simulating future outcomes, identifying risk, and uncovering uncertainty. Today, rapid changes in the industry have companies wanting more out of their analytic solutions, whereby the analytics take the next step to offer potential solutions to mitigate identified risk and uncertainty. This is where prescriptive analytics (or analytics that inform us of what should be done to improve the future) come into play.
A currently in-vogue type of prescriptive analytics is Machine Learning (ML). It is important to know that there are 3 general types of ML; unsupervised, supervised, and reinforced. For ML to offer potential solutions to a problem, this would fall under the category of reinforced learning, whereby a model or algorithm is set up to play a sort of problem-solving game. Over time in playing the game, the machine becomes smarter and smarter and is able to figure out optimal strategies to the problem and provide potential solutions.
One of the first places where reinforced ML manifested within the energy industry was in battery storage market participation. Bidding and delivering ancillary services and energy to the grid requires quick decision making, often within 5 minutes, to best leverage a battery. Inside this quick time frame, the amount of data that needs to be analyzed to make effective arbitrage decisions can exceed human capabilities.
Several companies are marketing ML solutions to meet this opportunity. As battery storage increases, this application will only become more important, which led to cQuant partnering with Fluence Software, a leader in Market Participation Software for batteries. As mentioned in Part One: Faster, Better, Stronger – Performance is Key, solution-specific platforms allow for greater focus and maintenance on the tasks the platform was designed to accomplish. cQuant, Fluence, and their strategic partnership is a great example of this philosophy.
Machine Learning is not the only technological path to prescriptive solutions. In fact, there are many instances where ML is not necessary or is even overkill. Advanced quantitative analytics, like ML can look into the future and provide solutions to a problem. ML is best used for super complex problems or when the aspect of competition is part of the problem.
cQuant.io is the first Energy Analytic Platform (EAP) to offer true Portfolio and Hedge Optimization, in which the cQuant model compares the possible choice for optimization to the organizations internal business goals and provides a rank ordered list of solutions. Although similar sounding to ML, this is accomplished through mathematical programming. That said, cQuant is always exploring use cases for Machine Learning as new problems enter the industry market.
To learn more about prescriptive analytics and how cQuant and our trusted partners can help your organization, click here to start a conversation with us.
About cQuant.io
cQuant.io is an industry leader in analytic solutions for energy and commodity companies. Specializing in Total Portfolio Analysis, cQuant’s cloud-native platform enables physical asset, financial contract, market simulation and risk management analytics in one place. cQuant is the leader in analytics for renewable, storage and other clean energy technologies. cQuant’s customers have greater insight into their financial forecasts and the drivers of value and risk in their business.