Retail Energy Analytics
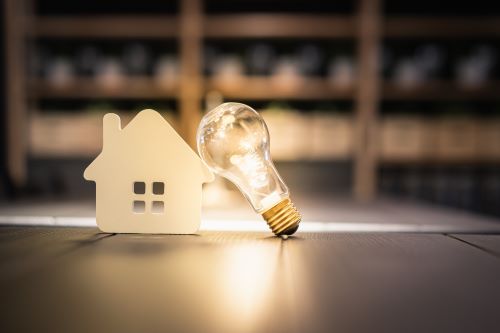
Business Questions
What is my expected retail energy revenue and how uncertain is this forecast?
How might changes in load shape and uncertainty due to behind-the-meter (BTM) solar and battery storage affect my portfolio?
How can I leverage sophisticated analytics to inform better rate design, particularly targeted at customers with BTM solar and battery storage?
How can I determine which customers are best/worst for my portfolio in terms of gross margin, revenue at risk, load shape, or other criteria?
How will acquiring a significant number of customers and associated demand affect my portfolio’s value and risk?
The Need for Analysis
Retail energy providers (REPs) often find themselves in the difficult position of being exposed to extreme volatility in their supply costs while receiving relatively static payments from the customers whose demand they serve. Additionally, customers are demanding “greener” and more sustainably generated electricity than ever before, forcing many retailers to procure intermittent renewable generation that cannot simply be dispatched when needed. All of this results in significant risk for the retailer, both in terms of volumetric demand uncertainty as well as the costs they must pay to satisfy unanticipated spikes in demand.
As the electricity grid becomes more and more decentralized, traditional retail energy paradigms are shifting. Changing wholesale price dynamics, increased behind-the-meter generation that robs valuable MWh from the portfolio, evolving regulatory environments, and service territory consolidation all add to the uncertainty about the future of retail energy, presenting significant challenges to retail portfolio managers. In many regions, the days of cheap and stable baseload energy are coming to an end.
Detailed analytics are needed to keep pace with evolving markets and portfolios. With retail rate structures becoming ever-more-dynamic, robust quantitative analytics can help to achieve a rare win-win with innovative rate designs providing financial benefit to both the retailer and the customer. In the midst of the “Energy Transition”, a solid quantitative understanding of retail energy portfolios is more important than ever.
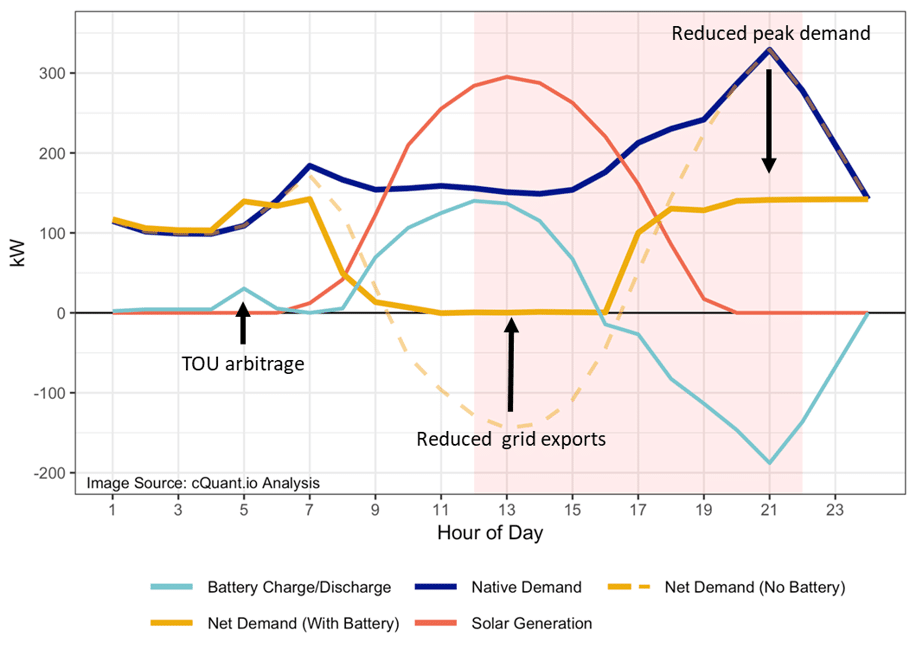
The cQuant.io Solution
cQuant’s retail energy analytics solution provides a comprehensive statistical description of retail portfolios today and into the future. Robust Monte Carlo simulation models for a variety of risk factors including demand, weather, market electricity prices (forward, spot, and basis), and renewable generation provide a framework within which users can understand not only expected future revenues but the uncertainty inherent in this forecast.
Support for a granular set of rate components including time-of-use schedules, demand charges, volumetric charges, feed-in tariffs, fixed charges, indexed rates, and others enables rapid scenario analysis and stress testing for rate design studies. Intuitive and flexible reporting allows insights to be achieved at the total portfolio level and also allows for “drill-down” into user-defined subportfolios to examine specific geographies, rate classes, customer types, or other aggregations.
cQuant’s retail analytics also integrate seamlessly with its Advanced Battery Storage Optimization model, allowing users to optimize batteries in behind-the-meter contexts for rate design or customer marketing purposes. With powerful analytics delivered in an intuitive user interface and backed by an infinitely-scalable cloud infrastructure, you can rest assured that you have the quantitative insight needed to manage your retail portfolio amid today’s every-changing energy landscape.
cQuant.io Users Can:
Compute value and risk for portfolios of retail energy contracts, viewing results at the total portfolio level or drilling down into user-defined subportfolios.
Report gross margin by customer class or schedule type, identifying subportfolios that are best or worst from a revenue and/or risk perspective.
Perform parametric analysis to isolate the financial impacts of changes in specific portfolio attributes such as hourly load shape, load uncertainty, wholesale market price, or increased distributed energy resource adoption.
Rapidly develop and test scenarios for rate design purposes, including behind-the-meter solar+storage systems and their response to various rate components.
Investigate the portfolio effects of service territory acquisitions or divestitures.